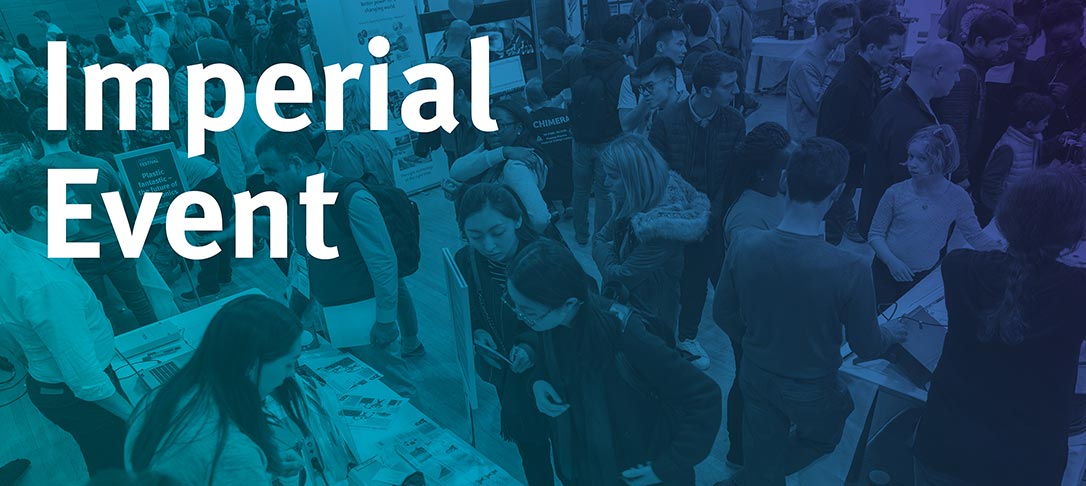
Talk Title
Distribution Generalization from a Causal Perspective
Talk Summary
The problem of Distribution Generalization (DG) is to learn a predictive function that works well when the test distribution differs from the training distribution. In recent years, DG has received much attention in machine learning (ML) applications sensitive to distributional shifts, such as healthcare or self-driving cars.
While several approaches to DG exist, recent research developed a framework for DG based on causality. In this tutorial, we dive into this framework to learn how to:
1. Formalize the DG setting as a robust optimization problem.
2. Describe the training and shifted test distributions with structural causal models (SCMs).
3. Show that causal models work well when the training and test distribution differ.
4. Estimate causal models from training data to achieve DG.
The tutorial will include hands-on examples with Jupyter notebooks in Python.
Speaker Bio – Dr Nicola Gnecco
Dr Nicola Gnecco is a visiting postdoctoral researcher in the Gatsby Computational Neuroscience Unit, working with Arthur Gretton and supported by a Swiss National Science Foundation research grant. Previously, he was a postdoctoral researcher at Yu Group, UC Berkeley, working with Bin Yu, and a postdoctoral researcher at Copenhagen Causality Lab, University of Copenhagen, working with Jonas Peters and Niklas Pfister. Nicola obtained a PhD supervised by Sebastian Engelke at the Research Center for Statistics, University of Geneva, and a master’s thesis in Statistics supervised by Nicolai Meinshausen at the Seminar for Statistics, ETH Zurich.
Time: 13.00 – 14.00
Date: Tuesday 8 October
Location: Hybrid Event | Online and in I-X Conference Room, Level 5
Translation and Innovation Hub (I-HUB)
Imperial White City Campus
84 Wood Lane
W12 0BZ
Link to join online via Teams.
Any questions, please contact Andreas Joergensen (a.joergensen@imperial.ac.uk).