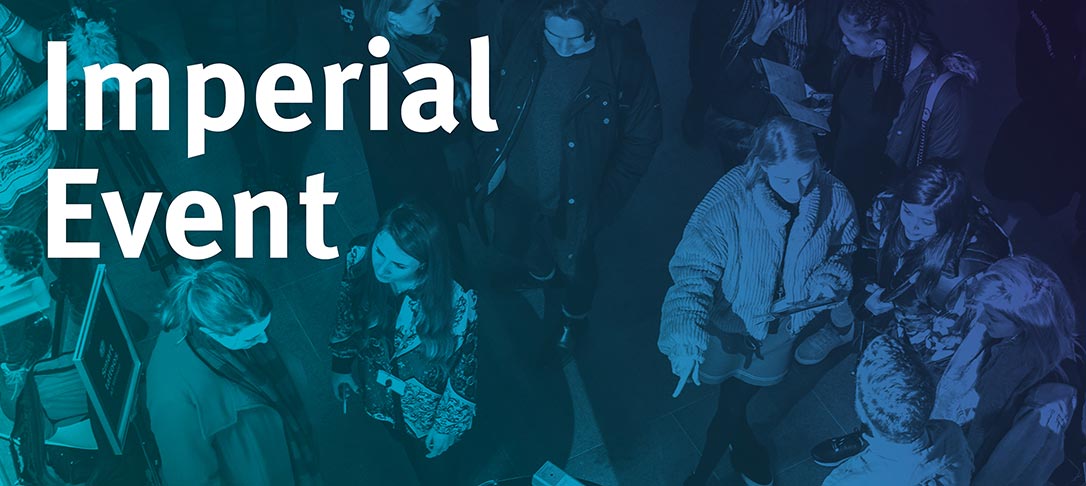
The main purpose of the London-Paris Bachelier Workshop on Mathematical Finance is strengthen cross-channel research collaborations by providing a unique in-person platform to exchange information about recent research developments in Mathematical Finance and related areas.
This seventh edition will take place in London on Monday 18 and Tuesday 19 September 2023 on the Imperial College campus.
It will focus on topics at the intersection of theory and applications, providing an important balance of the two, in particula covering recent advances in
Stochastic control,
Market microstructure,
Volatility modelling,
Machine learning in finance,
Climate finance,
Quantum Computing.
Local organisers: David Itkin, Ioannis Gasteratos.
Scientific commitee: Cyril Bénézet, Zorana Grbac, Antoine Jacquier and Jean-Francois Chassagneux, Cristopher Salvi, Almut Veraart.
Registration
Registration is free but mandatory for all participants. Please fill out the registration form available here. If additional information is required for registration we will contact you.
Schedule
Time | Monday Sep 18 |
9:45-10:00 | Coffee & Opening Remarks |
10:00-10:30 | Lukas Gonon |
10:30-11:00 | Caroline Hillairet |
11:00-11:15 | Alexandros Pavlis |
11:15-11:30 | Shaun Li |
11:30-12:00 | Andreas Sojmark |
12:00-13:00 | Lunch Break |
13:00-13:30 | Olivier David Zerbib |
13:30-13:45 | Ofelia Bonesini |
13:45-14:00 | Ioannis Gasteratos |
14:00-14:30 | Coffee Break |
14:30-15:00 | Kostas Kardaras |
15:00-15:30 | Sergio Pulido |
15:30-16:00 | Purba Das |
16:00-16:30 | Roxana Dumitrescu |
18:30 | Conference Dinner (By Invitation Only) |
Time | Tuesday Sep 19 |
9:45-10:00 | Coffee |
10:00-10:30 | Olivier Guéant |
10:30-11:00 | Luitgard Veraart |
11:00-11:15 | Zacharia Issa |
11:15-11:30 | Cecilia Aubrun |
11:30-12:00 | Alex Pannier |
12:00-13:00 | Lunch Break |
13:00-13:30 | Paul Gassiat |
13:30-13:45 | Mohamed Hamdouche |
13:45-14:00 | Nisrine Madhar |
14:00-14:30 | Coffee Break |
14:30-14:45 | Connor Tracy |
14:45-15:00 | Nicola Muca-Cirone |
15:00-15:30 | Neofytos Rodosthenous |
15:30-16:00 | |
16:00-16:30 | Yufei Zhang |
16:30 | Closing Remarks |
Titles and Abstracts
Cecilia Aubrun
Title: Classification of price jumps with wavelet coefficients
Abstract: Cascade events and extreme occurrences have garnered significant attention across diverse domains such as financial markets, seismology, and social physics. These events often stem from either the internal dynamics inherent to the system (endogenous) or external influences (exogenous). Distinguishing between these two event types holds critical implications for professionals in fields such as seismology, financial regulation, and marketing.
Prior research has established a key distinction between endogenous and exogenous events through their temporal asymmetry. Building upon this insight, we propose a novel unsupervised classification method for financial price jumps. This method leverages wavelet coefficients, which capture various aspects of signal asymmetry, including envelope asymmetry.
We introduce a 2D projection that not only characterizes the reflexive nature of price jumps but also quantifies their mean reversion tendencies. Through this representation, we identify three distinct classes of jumps: exogenous, endogenous, and anticipatory spikes.
Furthermore, by extending our univariate classification approach, we develop a methodology to investigate the reflexive properties of co-jumps, which occur when multiple stocks experience price jumps within the same minute. This comprehensive framework provides valuable insights into the dynamics of extreme events in financial markets and their underlying nature.
Ofelia Bonesini
Title: Weak rates of convergence for rough volatility models via PPDEs
Abstract: Option prices under rough volatility solve some path dependent PDEs, as a consequence of the functional Itô formula for Volterra processes. We exploit these results to investigate the weak rates of convergence for discretised stochastic integrals involving smooth functions of a Riemann-Liouville fractional Brownian motion with a Hurst parameter $H \in (0,1/2)$. These integrals serve as approximations for log-stock prices within rough volatility models. Our findings reveal that for quadratic test functions, we observe weak error rates of order $1$, while for smooth test functions, the rates are of order $H + 1/2$. This is a joint work with Prof. Jacquier and Ph.D. Pannier.
Purba Das
Title: Fake fractional Brownian motion
Abstract: We study how to construct a stochastic process on a finite interval with given `roughness’ and finite joint moments of marginal distributions. Our construction method is based on Schauder representation along a general non-uniform sequence of partitions. We show that the variation index of a process along a given partition sequence (the infimum value $p$ such that the $p$-th variation is finite) may not be equal to the reciprocal of H\”older exponent, and provide a pathwise estimator of H\”older exponent. Moreover, we construct a non-Gaussian family of stochastic processes which are statistically indistinguishable from (fractional) Brownian motions. Therefore, when observing a sample path from a process in a financial market such as a price or volatility process, we should not measure its H\”older roughness by computing $p$-th variation and should not conclude that the sample is from Brownian motion or fractional Brownian motion even though it exhibits the same properties of those Gaussian processes.
Roxana Dumitrescu
Title: A new Mertens decomposition]{A new $\mathcal{Y}^g$-Mertens decomposition and applications
Abstract: We first introduce the concept of $\mathscr{Y}^{g,\xi}$-submartingale systems, where the nonlinear operator $\mathscr{Y}^{g,\xi}$ corresponds to the first component of the solution of a reflected BSDE with generator $g$ and lower obstacle $\xi$. We first show that, in the case of a left-limited right-continuous obstacle, any $\mathscr{Y}^{g,\xi}$-submartingale system can be aggregated by a process which is right-lower semicontinuous. We then prove a \textit{Mertens decomposition}, by using an original approach which does not make use of the standard penalization technique. These results are in particular useful for the treatment of control/stopping game problems and, to the best of our knowledge, they are completely new in the literature. We present two applications to the partial hedging of American options and the pricing of American options in an incomplete market.
Paul Gassiat
Title: Zero noise limit for singular ODE regularized by fractional noise
Abstract: A classical manifestation of regularization by noise is that adding an irregular term to an ill-posed equation may restore well-posedness (existence/uniqueness). A natural question is then, in the limit where the coefficient in front of the noise is taken to zero, whether this selects one (or several) particular solutions to the original equation (this is typically referred to as “selection by noise”). In the case of one-dimensional ODEs, perturbed by a Brownian motion, Bafico and Baldi ’82 showed that this procedure selects extremal solutions, i.e. those that exit the problematic point instantly. We extend this result to the case of fractional noise (and obtain in addition some exponential concentration estimates). The main difficulty lies in the absence of the Markov property for the system. Our proof is based on the dynamical approach of Delarue-Flandoli ’14, combined with recent progress in regularisation by fractional noise (Catellier-Gubinelli ’16), and techniques coming from the study of ergodicity of fractional SDEs (Hairer ’05, Panloup-Richard ’20). Based on a joint work with Łukasz Mądry (Univ. Paris-Dauphine).
Ioannis Gasteratos
Title: Transportation-cost inequalities for rough-volatility models
Abstract: We consider a class of rough volatility models in which the volatility function is allowed to grow superlinearly and depends on a (non-Markovian) fractional Gaussian process. Using a generalized contraction principle, along with the regularity structure framework developed by Bayer, Friz, Gassiat, Martin and Stemper, we obtain transportation-cost inequalities for the law of nonlinear Gaussian functionals such as the stock log-price, Gaussian models and modelled distributions. The inequalities we study can be viewed as a functional approach to the concentration of measure phenomenon and are linked to exponential integrability and deviation estimates. If time permits, we shall discuss potential applications of our methodology to other examples of regularity structures. This is ongoing work with Antoine Jacquier.
Lukas Gonon
Title: Mathematical understanding of (random) neural networks for option pricing
Abstract: Deep learning algorithms have been shown empirically to work well in many classical problems from mathematical finance. Theoretical foundations of deep learning in this context, however, are far less developed. In this talk we present some recent results in this direction.
More specifically, the talk starts with examples of recent applications of deep learning related to option pricing. Next, we discuss theoretical foundations of such methods, recent mathematical progress, and key challenges. Finally, we show that in certain situations random neural networks are capable of overcoming these challenges.
Olivier Gueant
Title: Automated Market Makers: Mean-Variance Analysis of LPs Payoffs and Design of Pricing Functions
Abstract: With the emergence of decentralized finance, new trading mechanisms called Automated Market Makers have appeared. The most popular Automated Market Makers are Constant Function Market Makers. They have been studied both theoretically and empirically. In particular, the concept of im- permanent loss has emerged and explains part of the profit and loss of liquidity providers in Constant Function Market Makers. In this paper, we propose another mechanism in which price discovery does not solely rely on liquidity takers but also on an external exchange rate or price oracle. We also propose to compare the different mechanisms from the point of view of liquidity providers by using a mean / variance analysis of their profit and loss compared to that of agents holding assets outside of Automated Market Makers. In particular, inspired by Markowitz’ modern portfolio theory, we manage to obtain an efficient frontier for the performance of liquidity providers in the idealized case of a perfect oracle. Beyond that idealized case, we show that even when the oracle is lagged and in the presence of adverse selection by liquidity takers and systematic arbitrageurs, optimized oracle-based mechanisms perform better than popular Constant Function Market Makers.
Mohamed Hamdouche
Title: Generative Modeling for Time Series Via Schrödinger Bridge
Abstract: We propose a novel generative model for time series based on Schrödinger bridge (SB) approach. This consists in the entropic interpolation via optimal transport between a reference probability measure on path space and a target measure consistent with the joint data distribution of the time series. The resulting solution is described by a stochastic differential equation over a finite horizon with a path-dependent drift function, which accurately captures the temporal dynamics of the time series distribution. We estimate the drift function from data samples by kernel regression methods, which is particularly practical and computationally low-cost, and the simulation of the SB diffusion yields new synthetic data samples of the time series. The performance of our generative model is evaluated through a series of numerical experiments. This is a joint work with Pierre Henry-Labordere and Huyên Pham.
Caroline Hillairet
Title: Bi-revealed utilities in a defaultable universe: a new point of view on consumption
Abstract: In this talk, we investigate the inverse problem of bi-revealed utilities in a defaultable universe, defined as a standard universe (represented by a filtration F) perturbed by an exogenous defaultable time τ. We assume that the standard universe does not take into account the possibility of the default, thus τ adds an additional source of risk. The defaultable universe is represented by the filtration G up to time τ (τ included), where G stands for the progressive enlargement of F by τ. The basic assumption in force is that τ avoids F-stopping times. The bi-revealed problem consists in recovering a consistent dynamic utility from the observable characteristic of an agent. The general results on bi-revealed utilities, first given in a general and abstract framework, are translated in the defaultable G-universe and then are interpreted in the F-universe. The decomposition of G-adapted processes XG provides an interpretation of a G-characteristic XτG stopped at τ as a reserve process. Thanks to the characterization of G-martingales stopped at τ in terms of F-martingales, we establish a correspondence between G-bi-revealed utilities from characteristic and F-bi-revealed pair of utilities from characteristic and reserves. In a financial framework, characteristic can be interpreted as wealth and reserves as consumption. This result sheds a new light on the consumption in utility criterion: the consumption process can be interpreted as a certain quantity of wealth, or reserves, that are accumulated for the financing of losses at the default time. Based on a joint work with Nicole El Karoui and Mohamed Mad.
Zach Issa
Title: Non-adversarial training of Neural SDEs with signature kernel scores
Abstract: Neural SDEs are continuous-time generative models for sequential data. State-of-the-art performance for irregular time series generation has been previously obtained by training these models adversarially as GANs. However, as typical for GAN architectures, training is notoriously unstable, often suffers from mode collapse, and requires specialised techniques such as weight clipping and gradient penalty to mitigate these issues. In this paper, we introduce a novel class of scoring rules on pathspace based on signature kernels and use them as objective for training Neural SDEs non-adversarially. By showing strict properness of such kernel scores and consistency of the corresponding estimators, we provide existence and uniqueness guarantees for the minimiser. With this formulation, evaluating the generator-discriminator pair amounts to solving a system of linear path-dependent PDEs which allows for memory-efficient adjoint-based backpropagation. Moreover, because the proposed kernel scores are well-defined for paths with values in infinite dimensional spaces of functions, our framework can be easily extended to generate spatiotemporal data. Our procedure permits conditioning on a rich variety of market conditions and significantly outperforms alternative ways of training Neural SDEs on a variety of tasks including the simulation of rough volatility models, the conditional probabilistic forecasts of real-world forex pairs where the conditioning variable is an observed past trajectory, and the mesh-free generation of limit order book dynamics.
Kostas Kardaras
Title: Price Impact Under Heterogeneous Beliefs and Restricted Participation
Abstract: We consider a financial market in which traders potentially face restrictions in trading some of the available securities. Traders are heterogeneous with respect to their beliefs and risk profiles, and the market is assumed thin: traders strategically trade against their price impacts. We prove existence and uniqueness of a corresponding equilibrium, and provide an efficient algorithm to numerically obtain the equilibrium prices and allocations given market’s inputs. Surprisingly, we find that restrictions may increase the market’s welfare if traders have different views regarding the covariance matrix of the securities’ returns. (Joint work with Michail Anthropelos)
Shaun Li
Title: Joint SPX-VIX calibration: the quintic Ornstein Uhlenbeck volatility model
Abstract: The quintic Ornstein-Uhlenbeck (OU) volatility model is a stochastic volatility model where the volatility process is a polynomial function of degree five of a single OU process with fast mean reversion and large vol-of-vol. The model can achieve remarkable joint fits of the SPX-VIX smiles with only 6 effective parameters and an input curve that allows to match certain term structures. Even better, the model remains very simple and tractable for pricing and calibration: 1) the VIX squared is again polynomial in the OU process, leading to efficient VIX derivative pricing by a simple integration with respect to the Gaussian density; 2) the model is exponential affine (in infinite dimension), leading to fast pricing of SPX derivatives via the Fourier Laplace Transform characterized by a system of infinite dimensional Riccati ODEs. Based on joint works with Eduardo Abi Jaber (Ecole Polytechnique) and Camille Illand (AXA Investment Managers).
Nisrine Madhar
Title: Spanning Multi-Asset Payoffs With ReLUs
Abstract: Payoff functions of continuum portfolios of vanilla basket calls or puts can be seen as one hidden-layer feedforward ReLU neural networks, a mapping that can also be stated in terms of Radon transforms. The universal approximation theorem then implies that any multi-asset payoff can be approximated arbitrarily well on compact sets by finite portfolios of vanilla basket payoffs. This work specifies conditions under which the only possible solution to the continuous spanning inverse problem is the inverse Radon transform of the second derivative of the target payoff with respect to the strike variable. A numerical study demonstrates the practicality of related training schemes to the corresponding discrete spanning problem, spanning a selection of archetypal multi-asset payoffs using vanilla basket payoffs.
Nicola Muca-Cirone
Title: Rough Kernel Hedging
Abstract: We propose a new method to solve hedging problems using Kernels defined on sequential data. The technique is highly versatile being model agnostic, independent from any specific choice of kernel, and applicable across a wide range of convex hedging problems thanks to a powerful Representer Theorem result. Furthermore, it seamlessly applies to settings where the underlying assets evolve as semimartingales as well as more general weakly geometric rough paths.
Alexandre Pannier
Title: Path-dependent PDEs for rough volatility
Abstract: The functional Itô formula for Volterra processes implies that option prices under rough volatility solve path-dependent PDEs. This opens the gates to several applications. In this talk, we present weak rates of convergence, a numerical scheme based on signature kernels and a PDE for VIX options. These are joint works with O. Bonesini, A. Jacquier and C. Salvi.
Alexandros Pavlis
Title: An equilibrium model for optimal capacity expansion
Abstract: A singular control model for the capacity expansion of producers with CES demand function is introduced. Firstly, we discuss the case of a monopolist where closed form solutions for the value function as well as the optimal control are presented. Consequently, a mean field model of a representative agent, which arises as a natural scaling limit from the $N-$ producer model, is studied where the agent’s problem is also shown to possess closed form expressions
Sergio Pulido
Title: Understanding the worst-kept secret of high-frequency trading
Abstract: Volume imbalance in a limit order book is often considered as a reliable indicator for predicting future price moves. In this work, we seek to analyse the nuances of the relationship between prices and volume imbalance. To this end, we study a market-making problem which allows us to view the imbalance as an optimal response to price moves. In our model, there is an underlying efficient price driving the mid-price, which follows the model with uncertainty zones. A single market maker knows the underlying efficient price and consequently the probability of a mid-price jump in the future. She controls the volumes she quotes at the best bid and ask prices. Solving her optimization problem allows us to understand endogenously the price-imbalance connection and to confirm in particular that it is optimal to quote a predictive imbalance. The value function of the market maker’s control problem can be viewed as a family of functions, indexed by the level of the market maker’s inventory, solving a coupled system of PDEs. We show existence and uniqueness of classical solutions to this coupled system of equations. In the case of a continuous inventory, we also prove uniqueness of the market maker’s optimal control policy. This is joint work with Mathieu Rosenbaum and Emmanouil Sfendourakis.
Zhenjie Ren
Title: Uniform-in-time Propagation of Chaos for Mean-Field Langevin Dynamics
Abstract: In recent times, there has been a growing interest in the study of Mean-field Langevin (MFL) dynamics, primarily due to its natural application in training two-layer neural networks. To simulate the MFL dynamics’ invariant distribution, one relies on the corresponding $N$-particle system, hoping that the error between the particle system and the mean-field dynamics remains small over an extended period. Our recent research focuses on the uniform-in-time propagation of chaos for the MFL dynamics with convex mean-field potential, motivated by this observation. We establish that this holds true for the $L_2$-Wasserstein distance and relative entropy under mild conditions.
Neofytos Rodosthenous
Title: Non-zero-sum optimal stopping game with continuous versus periodic opportunities
Abstract: We introduce a new non-zero-sum game of optimal stopping with asymmetric exercise opportunities. Given a stochastic process modelling the value of an asset, one player observes and can act on the process continuously, while the other player can act on it only periodically at independent Poisson arrival times. The first one to stop receives a reward, different for each player, while the other one gets nothing. We study how each player balances the maximisation of gains against the maximisation of the likelihood of stopping before the opponent. In such a setup, driven by a Lévy process with positive jumps, we not only prove the existence, but also explicitly construct a Nash equilibrium.
Andreas Sojmark
Title: Distress contagion in a dynamic interbank model
Abstract: In this talk, I will introduce a stylized interbank model with stochastic dynamics and multiple maturities, in order to derive a systemic risk-aware term structure for interbank claims. This is done by enforcing a mark-to-market valuation adjustment, e.g. to account for informational contagion, through a forward-backward approach in which the conditional probabilities of future solvency are required to determine today’s balance sheets. The result of this exercise is a form of distress contagion that acts as a stochastic volatility term in the capital of each bank, leading, endogenously, to both volatility clustering and a marked downside `leverage effect’. Throughout, I will rely on simple numerical case studies to demonstrate the main financial implications.
Luitgard Veraart
Title: Systemic Risk in Markets with Multiple Central Counterparties
Abstract: We provide a framework for modelling risk and quantifying payment shortfalls in cleared markets with multiple central counterparties (CCPs). Building on the stylised fact that clearing membership is shared among CCPs, we develop a modelling framework that captures the interconnectedness of CCPs and clearing members. We illustrate stress transmission mechanisms using simple examples as well as empirical evidence based on calibrated data. Furthermore, we show how stress mitigation tools such as variation margin gains haircutting by one CCP can have spillover effects on other CCPs. The framework can be used to enhance CCP stress-testing, which currently relies on the “Cover 2” standard requiring CCPs to be able to withstand the default of their two largest clearing members. We show that who these two clearing members are can be significantly affected if one considers higher-order effects arising from interconnectedness through shared clearing membership. Looking at the full network of CCPs and shared clearing members is therefore important from a financial stability perspective. This is joint work with Iñaki Aldasoro (BIS).
David Olivier Zerbib
Title: When Green Investors Are Green Consumer
Abstract: We introduce investors with preferences for green assets to a general equilibrium setting in which they also prefer consuming green goods. Their preference for green goods induces consumption premia on expected returns, which counterbalance the green premium stemming from their preferences for green assets. Because they provide a hedge when green goods become expensive, brown assets command lower consumption premia, while green investors allocate a larger share of their portfolios towards them. Empirically, the green-minus-brown consumption premia differential reached 30-40 basis points annually and contributes to explaining the limited impact of green investing on the cost of capital of polluting firms.
Yufei Zhang
Title: Cost-effective learning for propagator models
Abstract: We consider a class of learning problems in which an agent liquidates a risky asset while creating both transient price impact driven by an unknown convolution propagator and linear temporary price impact with an unknown parameter. We characterize the trader’s performance as maximization of a revenue-risk functional, where the trader also exploits available information on a price predicting signal. We present a trading algorithm that alternates between exploration and exploitation phases and achieves sublinear regrets with high probability. For the exploration phase we propose a novel approach for nonparametric estimation of the price impact kernel by observing only the visible price process and derive sharp bounds on the convergence rate, which are characterised by the singularity of the propagator. The bound on the regret in the exploitation phase is obtained by deriving stability results for the optimizer and value function of the associated class of infinite-dimensional stochastic control problems.